The introduction of MobileLLM marks a significant advancement in the field of artificial intelligence, particularly in adapting complex language models for use on resource-constrained devices like smartphones. Published on June 27, 2024, by a team involving Meta Reality Labs, PyTorch, and Meta AI Research (FAIR), this innovation challenges the conventional belief that effective AI models require massive parameters.
Unlike models such as GPT-4, which can exceed a trillion parameters, MobileLLM focuses on efficiency with models containing fewer than 1 billion parameters.
Key innovations in MobileLLM include prioritizing model depth over width, implementing embedding sharing and grouped-query attention, and utilizing block-wise weight-sharing techniques.
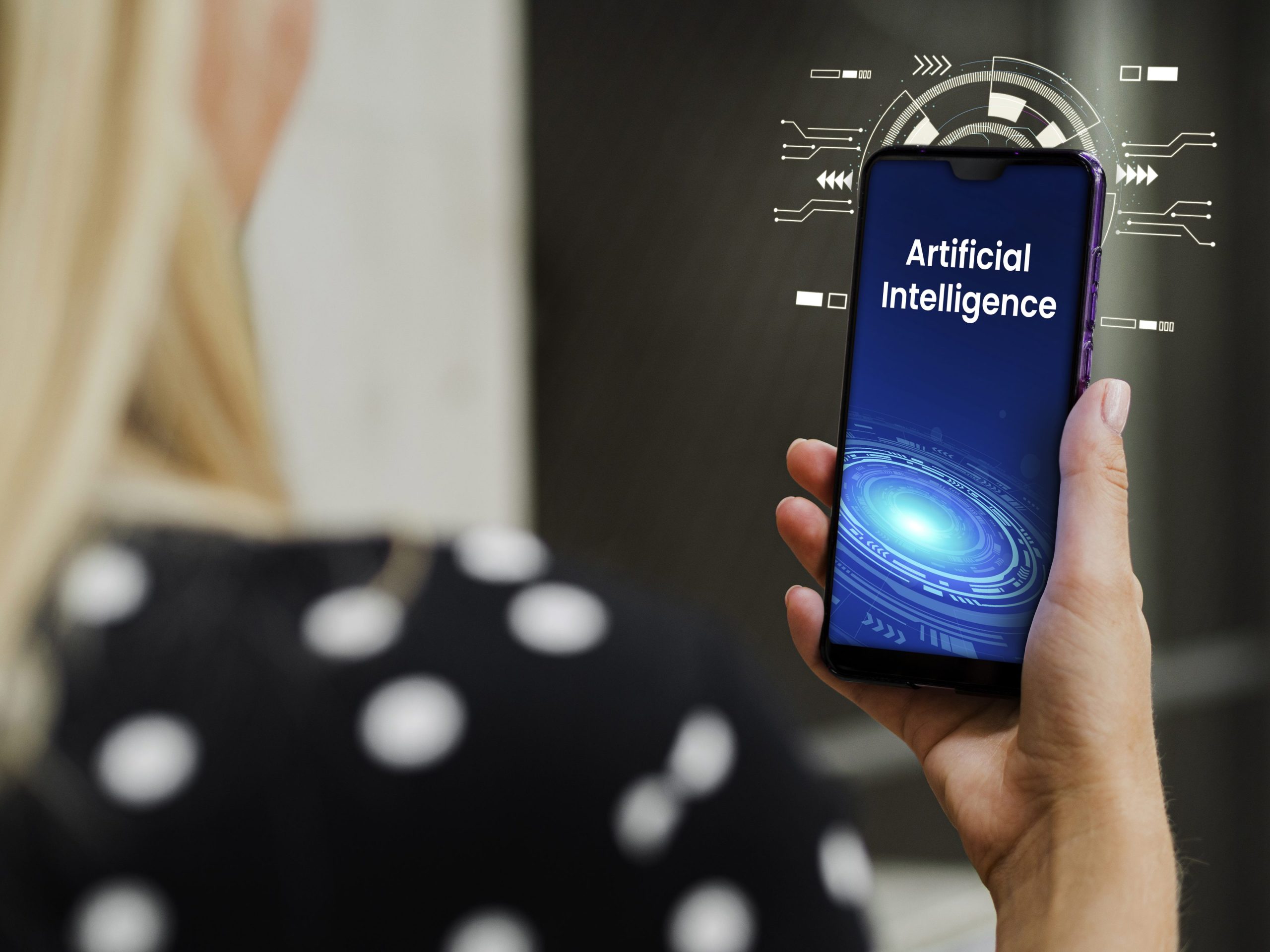
These design choices have enabled MobileLLM to achieve notable performance gains of 2.7% to 4.3% on standard benchmark tasks compared to previous models of similar size. This incremental improvement underscores the competitive edge gained through strategic model optimization.
One of MobileLLM’s noteworthy achievements is demonstrated by its 350 million parameter version, which achieved comparable accuracy in certain API calling tasks to the much larger 7 billion parameter LLaMA-2 model.
This suggests that compact models like MobileLLM could offer similar functionalities while consuming significantly fewer computational resources, making them highly suitable for on-device applications.
MobileLLM’s development aligns with a broader trend towards more efficient AI models, reflecting a shift away from the pursuit of ever-larger models. As interest in scalable and sustainable AI grows, MobileLLM’s open-sourced pre-training code allows researchers to build upon its innovations, potentially paving the way for advanced AI applications on personal devices in the future.
MobileLLM represents a pivotal advancement in AI accessibility and sustainability by challenging the necessity of immense model sizes. By optimizing for efficiency and on-device deployment, MobileLLM sets a precedent for future developments in compact yet powerful language models, promising broader integration of AI technologies into everyday personal devices.
Leave a Reply